Understanding the Significance of Image Annotation for Machine Learning
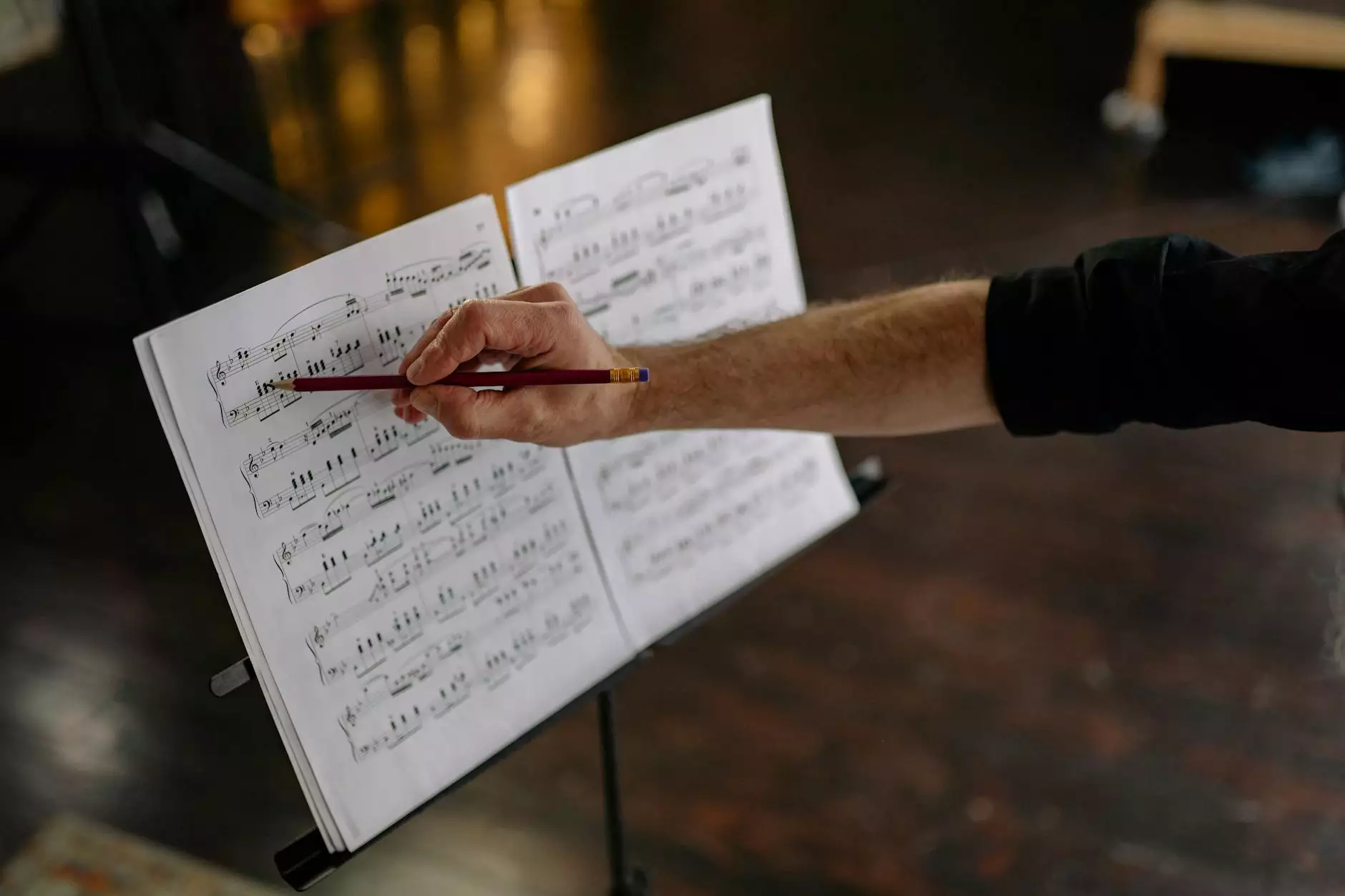
In the age of artificial intelligence (AI), machine learning (ML) has emerged as a crucial component for developing intelligent systems across various industries. A fundamental requirement for effective machine learning models is the availability of high-quality training data. One particularly vital aspect of this data preparation is image annotation. In this comprehensive article, we will delve into the importance of image annotation for machine learning, discussing its applications, techniques, and the solutions provided by KeyLabs AI.
The Role of Image Annotation in Machine Learning
Machine learning models rely on vast amounts of data to learn and make predictions. Image annotation refers to the process of labeling and tagging images to prepare them for training ML algorithms. This process is essential for various applications, including:
- Object Detection: Identifying and locating objects within images.
- Image Segmentation: Dividing an image into segments to simplify analysis.
- Facial Recognition: Recognizing and verifying individuals within images.
- Scene Understanding: Interpreting the elements present in a scene.
- Autonomous Vehicles: Assisting in real-time decision-making processes.
Each of these applications is crucial for advancing technologies such as computer vision, robotics, and even healthcare diagnostics.
Image Annotation Techniques
Image annotation employs various techniques to achieve different labeling objectives. Here are some of the most prevalent techniques:
Bounding Box Annotation
One of the simplest methods, bounding box annotation involves drawing rectangular boxes around objects in images. This method is widely used for object detection tasks and is integral to training models like YOLO (You Only Look Once) and SSD (Single Shot MultiBox Detector).
Polygon Annotation
Unlike bounding boxes, polygon annotation allows for more precise labeling of objects by outlining their actual shape. This technique is beneficial for irregularly shaped objects and is commonly used in applications such as self-driving cars and agricultural monitoring.
Semantic Segmentation
This technique involves classifying each pixel in an image into different categories. It aids in understanding the context of images and is especially useful for tasks that require scene understanding, such as urban planning and medical image analysis.
Instance Segmentation
Instance segmentation combines object detection and semantic segmentation, distinguishing between different instances of the same object class in an image. This technique is vital for advanced applications like robotic manipulation and fine-grained visual recognition.
Challenges in Image Annotation
Despite its significance, image annotation poses several challenges, including:
- Time-Consuming: Manual image annotation can be labor-intensive, especially for large datasets.
- Subjectivity in Labeling: Different annotators may interpret images differently, leading to inconsistencies in the dataset.
- Quality Assurance: Ensuring high-quality annotations requires thorough review processes.
To overcome these challenges, leveraging automated annotation tools and platforms can significantly enhance the efficiency and accuracy of the annotation process.
KeyLabs AI: Advanced Data Annotation Tools and Platforms
At KeyLabs AI, we offer cutting-edge solutions for image annotation that streamline the process and enhance the quality of training data. Our data annotation tools include:
AI-Powered Annotation Tools
Utilizing artificial intelligence, our tools automate routine annotation tasks, significantly reducing the time required to annotate large datasets. This automation not only saves time but also minimizes human error, ensuring high-quality outputs.
Collaborative Annotation Platform
KeyLabs AI provides a collaboration-friendly platform for teams. Multiple contributors can work on the same project, track changes, and maintain version control, ensuring seamless workflow and communication throughout the annotation process.
Quality Control Mechanisms
We recognize the importance of accuracy in image annotation. Our platform integrates robust quality control measures, including regular audits, consensus mechanisms among annotators, and the use of feedback loops to enhance the annotation quality over time.
Benefits of Image Annotation with KeyLabs AI
Implementing KeyLabs AI in your machine learning projects offers numerous benefits:
- Scalability: Our platform provides resources to accommodate projects of any scale, allowing businesses to grow without being limited by their annotation capabilities.
- Cost-Efficiency: By combining human intelligence with AI, you can reduce operational costs for data annotation while maintaining high standards of quality.
- Faster Turnaround Time: Speed up your project timelines with our advanced tools, enabling quicker access to labeled datasets.
- Comprehensive Support: KeyLabs AI offers dedicated customer support to assist you with any challenges you might face along the way.
Future Trends in Image Annotation for Machine Learning
The landscape of image annotation is evolving rapidly, and several trends are shaping its future:
Increased Use of Automated Tools
As AI technologies advance, the reliance on automated tools for image annotation is expected to grow. These tools will increasingly complement human annotators, leading to more efficient processes and better results.
Integration with Deep Learning Models
The synergy between annotation systems and deep learning models is becoming more pronounced. Future image annotation solutions will likely incorporate feedback from deep learning algorithms to refine annotation methods and improve overall accuracy.
Focus on Privacy and Ethics
With growing concerns regarding data privacy and ethical considerations in AI, future image annotation practices will need to address these issues. Companies will be required to implement strict guidelines and transparent practices in their data handling processes.
Conclusion
In conclusion, image annotation for machine learning is an indispensable component of modern AI applications. It enables the training of robust models that are crucial for tomorrow's innovations. By utilizing advanced data annotation tools and platforms such as those offered by KeyLabs AI, businesses can enhance their capabilities, improve the quality of their data, and ultimately drive success in their machine learning endeavors. Embracing these technologies will not only streamline processes but also empower organizations to leverage AI's full potential.
For more information about our data annotation tools and to explore how KeyLabs AI can support your machine learning projects, visit KeyLabs AI.