The Power of Image Dataset for Object Detection in Modern Business
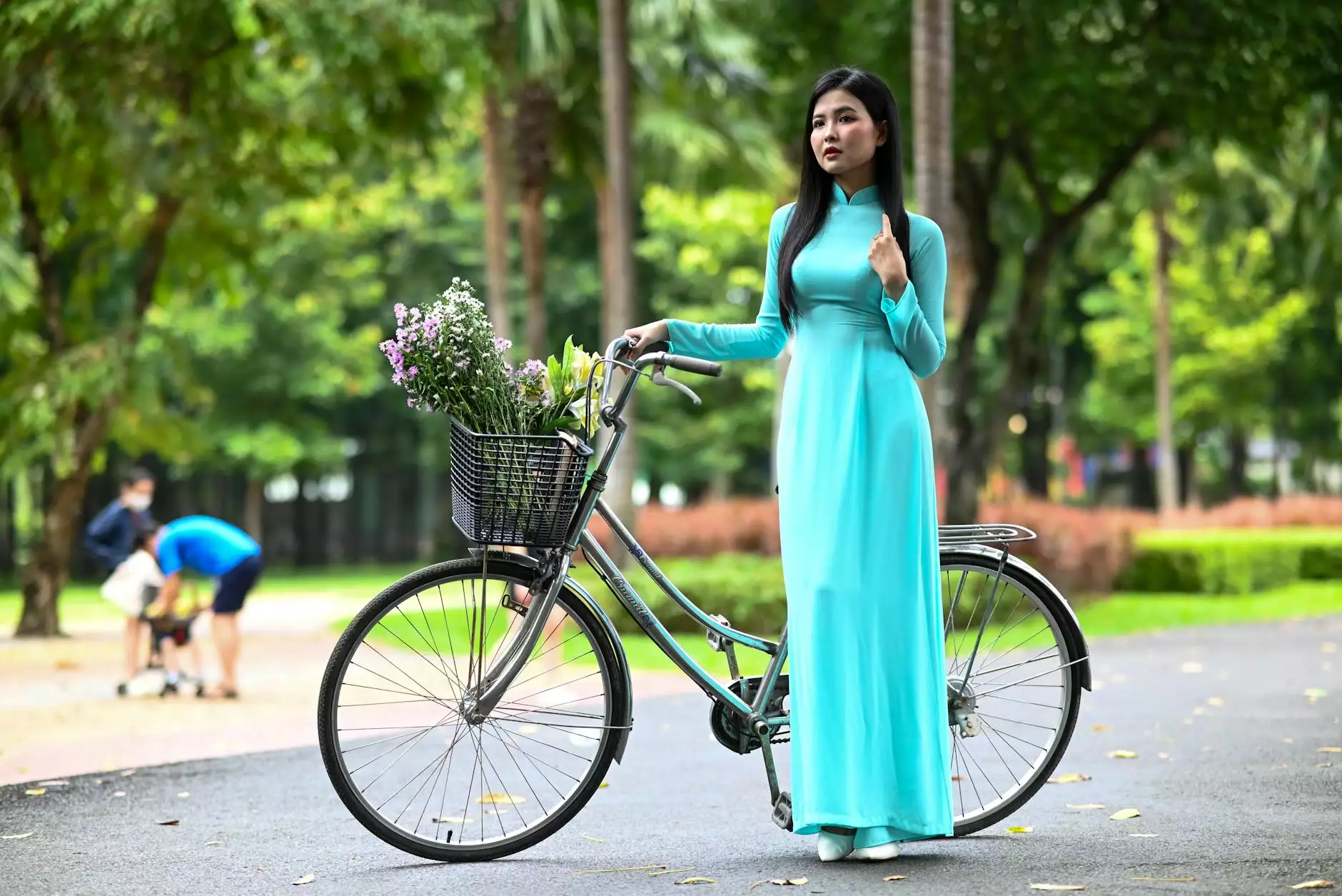
In the rapidly evolving landscape of technology, image datasets for object detection are becoming crucial for businesses aiming to leverage artificial intelligence and machine learning. Successfully implementing these technologies requires a clear understanding and effective use of data annotation tools and data annotation platforms. This article explores the significance, creation, and applications of image datasets, illustrating how keylabs.ai is at the forefront of this innovation.
What is Object Detection?
Object detection is a computer vision technique that involves identifying and locating objects within digital images. This process utilizes convolutional neural networks (CNNs) and various other algorithms to recognize patterns and features. The primary goal of object detection is to provide machines the ability to understand their visual environment, similar to how humans do.
The Role of Image Datasets in Object Detection
At the core of any object detection system lies the image dataset, which serves as the foundation for training and validating machine learning models. These large collections of labeled images enable algorithms to learn how to identify specific objects accurately. The importance of these datasets cannot be overstated, as they directly impact the model’s performance, accuracy, and reliability.
Key Components of Image Datasets
An effective image dataset should possess several key characteristics:
- Diversity: A well-rounded dataset includes a broad range of objects in various scenarios, lighting conditions, and backgrounds.
- Quality: High-resolution images with clear, precise annotations are vital for training accurate models.
- Annotation Accuracy: Each image must be labeled correctly to ensure that the model learns the right associations during training.
- Volume: A large quantity of images is necessary to create a robust dataset that can generalize well across different applications.
Creating Image Datasets for Object Detection
The creation of effective image datasets involves several important steps:
1. Data Collection
The first step in creating an image dataset for object detection involves data collection. This can be achieved through:
- Public Datasets: Utilizing already available datasets like COCO, PASCAL VOC, or ImageNet.
- Web Scraping: Collecting images from websites while ensuring that proper licensing and permissions are adhered to.
- In-house Data Creation: Capturing images using cameras and smartphones that specifically cater to the target objects.
2. Data Annotation
Once the images are collected, they must be annotated appropriately. This process involves:
- Bounding Boxes: Drawing rectangles around objects of interest within each image.
- Semantic Segmentation: Labeling each pixel with a corresponding class, useful for more detailed detection and understanding.
- Keypoint Annotation: Marking specific points on objects which may be crucial for tasks like pose detection.
3. Quality Assurance
Ensuring the quality and accuracy of the annotations is critical. This can involve peer reviews, validation processes, and automated quality checks.
Importance of Data Annotation Tools and Platforms
Data annotation tools and platforms play a pivotal role in creating image datasets for object detection. They streamline the annotation process, enhance collaboration, and improve overall efficiency. Keylabs.ai provides top-tier solutions that make these processes easier and more effective.
Key Features of Effective Data Annotation Tools
- User-Friendly Interface: Intuitive design makes it easy for annotators to interact with the software.
- Collaboration Capabilities: Ability to work with teams effectively, allowing multiple users to sync their efforts.
- Multiple Annotation Types: Supports various annotation formats, including bounding boxes, polygons, and masks.
- Automated Tools: AI-assisted functionalities that can suggest annotations or automate repetitive tasks.
Applications of Object Detection in Business
Object detection has numerous applications across various industries, enhancing productivity and enabling innovative solutions. Here are some notable examples:
1. Retail and Inventory Management
Many retailers use object detection to monitor stock levels and optimize inventory management. AI-based cameras can analyze shelves in real-time, ensuring products are appropriately stocked, thus reducing losses due to out-of-stock scenarios.
2. Autonomous Vehicles
Self-driving cars rely heavily on object detection systems to identify vehicles, pedestrians, road signs, and other critical elements in their environment. This technology is essential for safe navigation and accident prevention.
3. Security and Surveillance
In the security sector, object detection aids in the identification of suspicious behavior or unauthorized intrusions by monitoring live feeds and alerting personnel when anomalies are detected.
4. Healthcare
In healthcare, object detection is used for identifying anomalies in medical imaging, such as tumors in X-rays or MRIs, thus enabling quicker and more accurate diagnoses.
Challenges in Creating Image Datasets for Object Detection
While the importance of image datasets for object detection is clear, the process is not without challenges. Some of these challenges include:
- Data Privacy Concerns: Data collection must be conducted ethically, ensuring compliance with regulations and standards.
- Lack of Diversity: An underrepresentation of certain classes can lead to biased models that perform poorly across various scenarios.
- Resource Intensity: Annotating datasets can be labor-intensive and time-consuming, demanding both skilled labor and funding.
Future Trends in Object Detection and Image Datasets
As technology advances, several trends are shaping the future of image datasets for object detection:
1. Enhanced Automation
Automation in data annotation, driven by AI advancements, will increasingly reduce the manual labor involved, allowing for quicker dataset generation and reducing costs.
2. Synthetic Data Generation
The rise of synthetic data generation allows businesses to create high-quality annotated datasets without the need for extensive photography or real-world trials, significantly cutting down on biases and enhancing diversity.
3. Continuous Learning Models
Future object detection systems will likely utilize continuous learning, enabling models to adapt and improve as they encounter new data, thus continuously enhancing accuracy over time.
Getting Started with Keylabs.ai
Businesses that wish to harness the power of image datasets for object detection should consider leveraging the services offered by keylabs.ai. The platform provides robust data annotation tools and platforms that streamline the entire process, ensuring high-quality datasets tailored to specific business needs.
- Custom Solutions: Keylabs.ai offers flexible solutions tailored to your unique business requirements.
- Expert Support: Their team of experts provides guidance and support to ensure the best outcomes for your projects.
- Scalability: Keylabs.ai's solutions are designed to scale with your business needs, accommodating projects of any size.
Conclusion
In conclusion, the significance of image datasets for object detection in business cannot be overstated. By understanding the intricacies involved in creating these datasets and using effective data annotation tools, companies can harness the power of AI and machine learning to create groundbreaking solutions. With keylabs.ai, organizations can pave the way for innovation and stay ahead of the competition in their respective fields.