Unlocking the Future: The Importance of Video Annotation Tools for Machine Learning
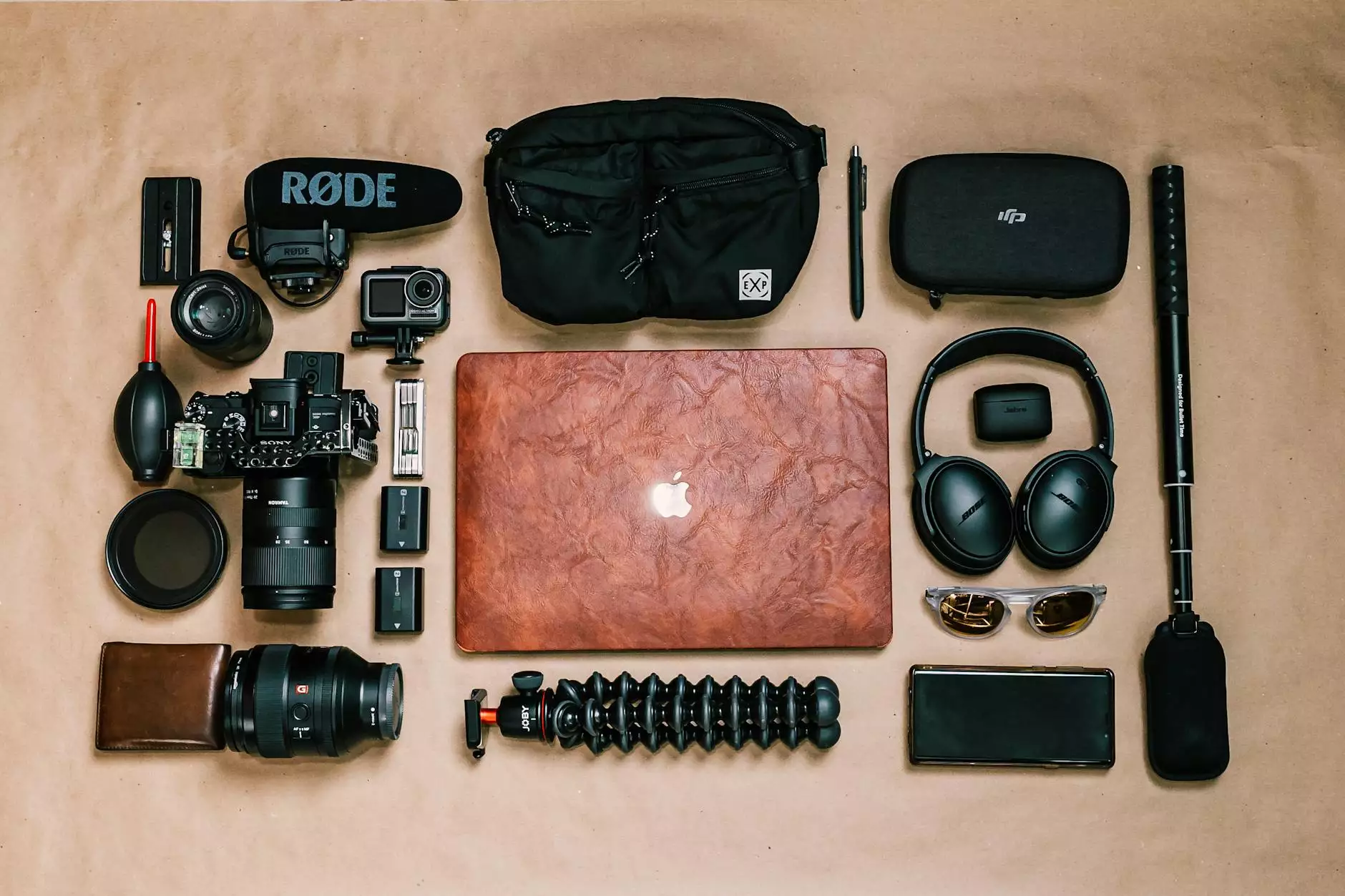
As we delve deeper into the era of artificial intelligence and machine learning, the significance of high-quality data has never been more pronounced. One of the pivotal aspects of data preparation is video annotation, a process that involves labeling video data to train machine learning models effectively. In this article, we will explore the critical role of video annotation tools for machine learning in enhancing various applications across numerous industries.
The Emergence of Machine Learning and Computer Vision
Machine learning has revolutionized how machines interpret data, particularly in the realm of computer vision. With the advent of technology, countless applications have emerged, enabling machines to “see” and understand the world. From autonomous vehicles to real-time surveillance systems, the ability to process video data efficiently is crucial. Video annotation tools for machine learning equip data scientists and engineers with the means to teach machines how to recognize patterns within video content.
What is Video Annotation?
Video annotation is the process of adding informative labels to video data, allowing machine learning algorithms to learn from this data. Labels can include identifying objects, segments of action, key activities, or emotional nuances depicted in the footage. This meticulous labeling process is essential for training algorithms to accurately predict and classify video content.
Why Use Video Annotation Tools?
Utilizing dedicated video annotation tools for machine learning offers several advantages:
- Efficiency: Automated tools can significantly reduce the time required for annotation, making it feasible to process large datasets.
- Accuracy: Advanced tools integrate machine learning assistance, enhancing the precision of annotations.
- Team Collaboration: Many annotation platforms facilitate a collaborative environment, allowing multiple annotators to work efficiently on the same project.
- Real-time Feedback: Certain tools provide immediate validation, ensuring that annotations meet the required standards.
The Components of Effective Video Annotation Tools
A high-quality video annotation tool for machine learning should encapsulate several vital features:
1. User-Friendly Interface
The interface of an annotation tool should be intuitive and straightforward, allowing users to focus on the annotation tasks without navigating through complex menus. A user-friendly interface minimizes training time and fosters productivity.
2. Versatile Annotation Types
Whether it’s object detection, action recognition, or event tagging, a versatile tool that supports various annotation types enhances flexibility. Users can choose the appropriate annotation type based on their project’s requirements.
3. Integration Capabilities
Seamless integration with existing machine learning frameworks and tools is essential. This feature allows for a streamlined workflow, enabling efficient data transfer and model training.
4. Quality Control Measures
An effective annotation tool includes mechanisms for quality control to ensure that annotations are both accurate and consistent. Implementing reviewer workflows can help maintain the integrity of the annotated data.
5. Scalability
As projects grow, the annotation tool must scale accordingly. The ability to handle increased data volume without sacrificing performance is crucial for long-term use.
Applications of Video Annotation in Machine Learning
The applications of video annotation tools for machine learning are extensive and varied:
1. Autonomous Vehicles
Autonomous driving technology relies heavily on video annotation to identify pedestrians, road signs, and other vehicles. Accurate video annotation facilitates the development of algorithms that enhance safety and navigation systems for autonomous vehicles.
2. Healthcare
In the healthcare sector, video annotation assists in analyzing medical imagery and video data, enabling early diagnosis and treatment. By labeling critical elements in videos, healthcare professionals can train models to detect anomalies, such as tumors in MRI scans.
3. Security and Surveillance
Video surveillance systems utilize machine learning to identify suspicious behavior or incidents. Annotating video feeds helps in training models to recognize security threats in real-time, improving response times and enhancing public safety.
4. Sports Analytics
In sports, video annotation is used to analyze player movements, strategies, and game dynamics. By annotating game footage, teams can gain insights into performance, helping coaches develop better training plans and strategies.
5. Retail and Customer Insights
Retailers can leverage video annotation to analyze customer behavior in stores. By annotating video surveillance data, businesses can identify traffic patterns, peak shopping times, and customer interactions, leading to improved store layout and customer service.
Choosing the Right Video Annotation Tool
When selecting a video annotation tool for machine learning, consider the following aspects:
- Project Requirements: Assess the specific needs of your project, such as the type of video content, required annotations, and the scale of work.
- Budget: Determine your budget for the annotation tool while considering the long-term return on investment.
- Support and Training: Ensure that the tool provider offers adequate support and training resources to help your team get up to speed.
- User Feedback: Look for reviews and testimonials from other users to gauge the tool’s reliability and effectiveness.
Best Practices for Video Annotation
To maximize the effectiveness of your annotation efforts, following best practices is essential:
1. Define Clear Annotation Guidelines
Before starting the annotation process, establish a set of guidelines that outline how annotations should be performed. Consistency in labeling is crucial to ensure high-quality data.
2. Train Your Annotators
Invest time in training your annotators to ensure they understand the intricacies of the project and the guidelines. Well-trained annotators are more likely to produce accurate and reliable results.
3. Regularly Review Annotations
Implement a quality assurance process where annotations are regularly reviewed for accuracy. This feedback loop helps identify and rectify issues early in the process, improving overall data quality.
4. Leverage Automated Tools
Utilize AI-assisted annotation features when available, as they can significantly speed up the process while maintaining a high standard of accuracy.
5. Keep the End Goal in Mind
Always remain focused on how the annotated data will be used. Understanding the end goal of the project will help in making more informed decisions during the annotation process.
The Future of Video Annotation in Machine Learning
The future of video annotation tools for machine learning is bright and full of potential. As technology continues to advance, we can expect:
- Increased Automation: The integration of more sophisticated AI technologies will automate even more aspects of the annotation process.
- Real-Time Annotation: Future tools may offer real-time annotation capabilities, drastically reducing turnaround times for projects.
- Cross-Platform Compatibility: Enhanced interoperability between different machine learning platforms and annotation tools is likely to emerge, streamlining workflows.
- Enhanced Collaboration Features: Future tools will likely focus on collaboration, allowing teams to work together seamlessly across geographical boundaries.
Conclusion
In conclusion, the utilization of video annotation tools for machine learning is critical for organizations looking to harness the power of video data. These tools enhance the quality of labeled data and drive the performance of machine learning models across various industries. As we continue to witness advancements in technology, the capabilities of video annotation tools will expand, enabling even more innovative applications in the realm of machine learning.
To explore the best video annotation platform, visit KeyLabs.ai, where you can find robust solutions tailored to meet your organization's needs.